Number Of Slots In Hash Table
Posted : admin On 4/3/202210.2.1. Hash Function Principles¶
Hashing generally takes records whose key values come from alarge range and stores those records in a tablewith a relatively small number of slots.Collisions occur when two records hash to the same slot in thetable.If we are careful—or lucky—when selecting a hash function,then the actual number of collisions will be few.Unfortunately, even under the best of circumstances, collisions arenearly unavoidable.To illustrate, consider a classroom full of students.What is the probability that some pair of studentsshares the same birthday (i.e., the same day of the year, notnecessarily the same year)?If there are 23 students, then the odds are about even that two willshare a birthday.This is despite the fact that there are 365 days in which studentscan have birthdays (ignoring leap years).On most days, no student in the class has a birthday.With more students, the probability of a shared birthday increases.The mapping of students to days based on their birthday is similar toassigning records to slots in a table (of size 365) using thebirthday as a hash function.Note that this observation tells us nothing about whichstudents share a birthday, or on which days of the year sharedbirthdays fall.
The difference between using a good hash function and a bad hash function makes a big difference in practice in the number of records that must be examined when searching or inserting to the table. Technically, any function that maps all possible key values to a slot in the hash table is a hash function. Demonstrate what happens when we insert the keys 5, 28, 19, 15, 20, 33, 12, 17, 10 5,28,19,15,20,33,12,17,10 into a hash table with collisions resolved by chaining. Let the table have 9 9 slots, and let the hash function be. Hash Tables 9/26/2019 15 29 Load Factor of a Hash Table Load factor of a hash table T: = n/N n = # of elements stored in the table N = # of slots in the table = # of linked lists is the average number of elements stored in a chain can be 1 0 N -1 T chain chain chain chain 30 Case 1:Unsuccessful Search. The number of items mapped to slot 1 is therefore X = X1 + X2 +.+Xn. The expected value of Xi is 1 k, for each i. Hence, the expected number of items mapped to slot 1 is E(X) = Xn i=1 E(Xi) = n k. But this is obvious in any case. As mentioned earlier, the expected numberof items is the same for every slot. Writ-ing Yj for the number of items.
Try it for yourself.You can use the calculator to see the probability of a collision.The default values are set to show the number of people in a room suchthat the chance of a duplicate is just over 50%.But you can set any table size and any number of records to determinethe probability of a collision under those conditions.
Use the calculator to answer the following questions.
To be practical, a database organized by hashing must store records in ahash table that is not so large that it wastes space.To balance time and space efficiency, this means that the hash tableshould be around half full.Because collisions are extremely likely to occur under these conditions(by chance, any record inserted into a table that is half full shouldhave a collision half of the time),does this mean that we need not worry about how well a hash functiondoes at avoiding collisions?Absolutely not.The difference between using a good hash function and a bad hash functionmakes a big difference in practice in the number of records that must beexamined when searching or inserting to the table.Technically, any function that maps all possible key values to aslot in the hash table is a hash function.In the extreme case, even a function that maps all records to the sameslot in the array is a hash function, but it does nothing to help usfind records during a search operation.
We would like to pick a hash function that maps keysto slots in a way that makes each slot in the hash table have equalprobablility of being filled for the actual set keys being used.Unfortunately, we normally have no control over the distribution ofkey values for the actual records in a given database or collection.So how well any particular hash function doesdepends on the actual distribution of the keys used within theallowable key range.In some cases, incoming data are well distributed across their keyrange.For example, if the input is a set of random numbers selecteduniformly from the key range,any hash function that assigns the key range so that each slot in thehash table receives an equal share of the range will likely alsodistribute the input records uniformly within the table.However, in many applications the incoming records are highlyclustered or otherwise poorly distributed.When input records are not well distributed throughout the key rangeit can be difficult to devise a hash function that does a good job ofdistributing the records throughout the table, especially if theinput distribution is not known in advance.
There are many reasons why data values might be poorly distributed.
- Natural frequency distributions tend to follow a common pattern wherea few of the entities occur frequently while most entities occurrelatively rarely.For example, consider the populations of the 100 largest cities inthe United States.If you plot these populations on a numberline, most of themwill be clustered toward the low side, with a fewoutliers on the high side.This is an example of a Zipf distribution.Viewed the other way, the home town for a given person is far morelikely to be a particular large city than a particular small town.
- Collected data are likely to be skewed in some way.Field samples might be rounded to, say, thenearest 5 (i.e., all numbers end in 5 or 0).
- If the input is a collection of common English words, the beginningletter will be poorly distributed.
Note that for items 2 and 3 on this list,either high- or low-order bits of the key are poorly distributed.
When designing hash functions, we are generally faced with one of twosituations:
- We know nothing about the distribution of the incoming keys.In this case, we wish to select a hash function that evenlydistributes the key range across the hash table,while avoiding obvious opportunities for clustering such as hashfunctions that are sensitive to the high- or low-order bits of the keyvalue.
- We know something about the distribution of the incoming keys.In this case, we should use a distribution-dependent hash functionthat avoids assigning clusters of related key values to the same hashtable slot.For example, if hashing English words, we should not hash onthe value of the first character because this is likely to be unevenlydistributed.
In the next module, you will see several examples of hash functionsthat illustrate these points.
Though using an Array, we can search an element with time complexity O(1), but the array has its limitation such as it stores similar data types, each cell of array occupies the same amount of space and to find an element we require its index value. To find the Index value of an element itself can take a time complexity of O(n) or O(log n).
Using the concept of Hashing we can build a data structure that can search elements with constant time complexity.
What is Hashing?
Hashing is a Technique in which we store data, in an array, at specific indices using some methods, rather than then storing it in ascending order, descending order or randomly. Suppose if we want to store 4 in an array, we perform some methods or operations on 4 and calculate the perfect index value for it, and if we want to retrieve 4 from the array, we just reverse that method or operation and get 4 with constant time complexity.
Hashing Table
Hashing Table or Hash Table is a collection of elements which are stored in a data structure using a Hashing method, which makes it easy to find them later. The Hash table consists of key and index or slot, here key represents the value which will store in the table and index or slot represent the index location of that key.
Each position of the hash table, slots, can hold an item and is named by an integer value starting at 0. We can use an Array to implement a hash table and initially all the elements of the array would be None.
For Example:
Insert 1, 3 ,5 , 7 , 8, 10, 11 in a hash table using hashing.
Create an array arbitrary size
Key-Value | Hashing (key value % size of array) | Array Index |
1 | 1 % 7 = | 1 |
3 | 3 % 7 = | 3 |
5 | 5 % 7 = | 5 |
7 | 7 % 7 = | 0 |
9 | 9 % 7 = | 2 |
10 | 10 % 7 = | 3 (collision) 3 is already occupied Collision resolution = 3+1= 4 |
11 | 11 % 7 = | 4 (Collison) = 4+1=5(collision)= 5+1 = 6 |
Array will be arr = [7, 1, 9, 3, 10, 5, 11, None, None, None, None, None, None]
Hashing Function
Hash function, are also known as Hashing methods and they are used to map each element or key to a unique slot or index. Hash is also used to minimize the number of collisions and using some easy methods it computes and evenly distributes the items in the hash table.
There are various hashing methods we can use to map a key to its slot:
- Remainder Method
- Folding Method
- Mid Square Method
1. Reminder method
In the reminder method, we use the divide the key value with the total size of the table or array and use it remainder to specify the index or slot value of that key.
For example, if we want to insert 9 in an array of size 20, so it would be placed at 9%20 = 9th index if 9th index is free.
2. Folding Method
The folding method for constructing hash functions begins by dividing the item into equal-size pieces (the last piece may not be of equal size).
These pieces are then added together to give the resulting hash value.
This hashing method applies to large digit numbers, for example, if we want a hash table in which key elements are the mobile numbers of the customers, this reminder method would not be efficient.
Number Of Slots In Hash Tableau
For instance:
If our key was the phone number 436-555-4601
We would take the digits and divide them into groups of 2 (43,65,55,46,01).
After the addition, 43+65+55+46+01, we get 210.
If we assume our hash table has 11 slots, then we need to perform the extra step of dividing by 11 and keeping the remainder.
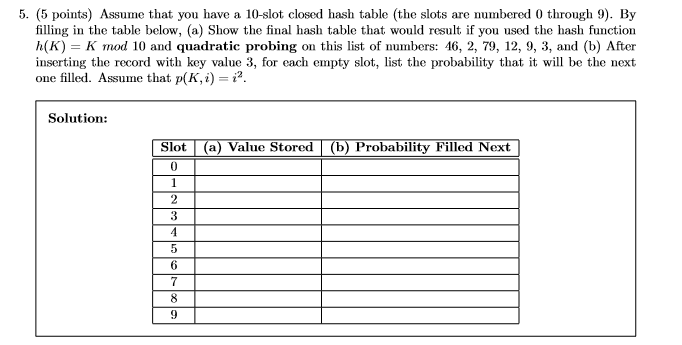
210 % 11 is 1, so the phone number 436-555-4601 hashes to slot 1.

Number Of Slots In Hash Tablespoon
3. Mid Square Method

In the mid-square method, we to compute the key slot number or index location we first square the item and then extract some portion of the resulting digits.
For example:
if the item were 44, we would first compute 442=1,936.
By extracting the middle two digits, 93, and performing the remainder step, we get 93%11 = 5
Number Of Slots In Hash Tables
Implementation of Hash table
Python
Output: